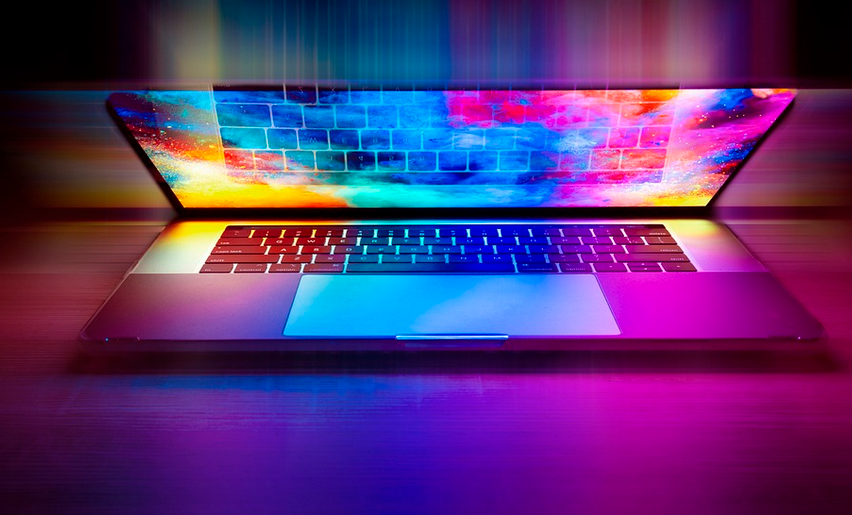
The Heart of Good Science
Imagine you’re working on a research project, pouring your heart and soul into analyzing data that promises to revolutionize our understanding of the world. But wait, there’s a catch—the data is messy! It’s full of inconsistencies, missing values, and outliers that threaten to derail your entire study. This is where data quality comes in, becoming the very foundation for reliable research.
Data quality isn’t just about creating pretty graphs or numbers; it’s about ensuring that your findings are accurate, meaningful, and ultimately reproducible. It’s about building a solid foundation upon which you can confidently stand and argue your research’s value in the scientific community. So how do we achieve this elusive data goldmine?
Taking Your Data on a Quality Journey
Let’s dive into some essential steps to ensure your research data is sparkling clean and ready for prime time.
**1. Defining the Scope & Setting Up Clear Expectations:** Before you even start collecting data, take a moment to clarify your objectives. What questions are you trying to answer? What specific information do you need from your participants or subjects?
Establishing clear goals helps ensure all aspects of the research process—from data collection to analysis and interpretation—align with these fundamental tenets. This clarity prevents confusion, minimizes errors, and allows for efficient allocation of resources.
**2. The Art of Data Collection: A Game of Accuracy & Consistency:**
Data quality starts right from the outset when you’re collecting it. Think about your methods! Are you using online surveys? Conducting interviews? Or perhaps gathering physical samples? Whatever method you choose, ensure its robustness and precision. For instance, if employing a survey:
– Use clear and concise questions that are easy to understand.
– Experiment with different question formats (e.g., multiple-choice, Likert scales) to see what resonates best with your sample group.
– Ensure all respondents have access to the survey platform.
Cleaning Up Our Mess: Data Preprocessing
Data cleaning isn’t just about fixing errors; it’s about making data usable and ensuring that your analysis is as accurate as possible.
**3. The Power of Cleaning & Transforming:**
Think of this step like decluttering your room—but with more precision! You need to identify, correct, or remove any inconsistencies and errors within your dataset. Here’s how:
- **Handling Missing Data:** Missing values can be a pain in the neck. There are various techniques you can use to address them, such as imputation (filling in missing values with an estimated value) or deletion (removing cases with missing information). The best approach depends on the nature of your data and the research question.
- **Standardizing Variables:** Ensure consistency across different variables. Consider using a standardized format for dates, units, and scales to prevent inconsistencies that might lead to misinterpretations and biased analysis.
- **Data Transformation:** If your data needs some “tweaked” action, consider mathematical transformations like logarithmic or square root functions to normalize the data, enabling better statistical analyses.
Ensuring Data Integrity: The Importance of Verification
After cleaning your dataset, it’s vital to double-check everything you’ve done for accuracy. This step is where the “proofreading” aspect comes in.
**4. Double, Triple Check – Data Validation:**
Data validation ensures that all data points are consistent with each other and meet your research objectives. There are several ways to validate your data:
* **Cross-check against source material:** Verify your data with the original sources or any supporting documents for accuracy. * **Use statistical checks:** Employ techniques like correlation analysis, t-tests, or regression analysis to identify potential outliers that might have been missed in manual inspection. * **Run sensitivity analyses:** Evaluate how your results change when you introduce different assumptions and explore alternative scenarios.
Sharing Knowledge: Documenting the Journey
Finally, remember that sharing data is about more than just handing over the raw numbers; it’s about building trust and transparency in your research findings.
**5. Documentation Is Your Friend:**
Document everything! This isn’t just about keeping a record of the steps you took—it’s also about explaining exactly how the data was collected, cleaned, analyzed, and interpreted. This ensures that your work can be easily replicated by others. When you’re ready to share your research, ensure all methods, materials, codes, and analysis are clearly documented in a separate “research report” document.
**6. Sharing is Caring:**
Sharing your data with the scientific community fosters collaboration, reproducibility, and ultimately helps advance knowledge. Remember to share your data openly, adhering to any relevant policies or guidelines.